# Understanding Facebook's Algorithm: Friend or Foe?
Written on
Chapter 1: Facebook's Algorithm Exposed
In a recent discussion, Nick Clegg, Facebook’s head of global affairs, addressed the nature of personal feeds on the platform. He emphasized that while critics claim sensationalism dominates, the truth is many top posts are lighthearted and share positive themes. Clegg noted that a popular post featured a mother bear with her cubs crossing a street. This perspective aims to present a cheerful image of what users encounter on their feeds.
This paragraph will result in an indented block of text, typically used for quoting other text.
Section 1.1: The Mechanics of the News Feed
Clegg's explanation simplifies the complex technology behind Facebook's News Feed. He attributes much of the responsibility for what users see to them, stating that thousands of signals are analyzed, including the nature of the post, its popularity, and the device used. The algorithm's goal is to predict how relevant a post might be to an individual, based on the likelihood of interaction.
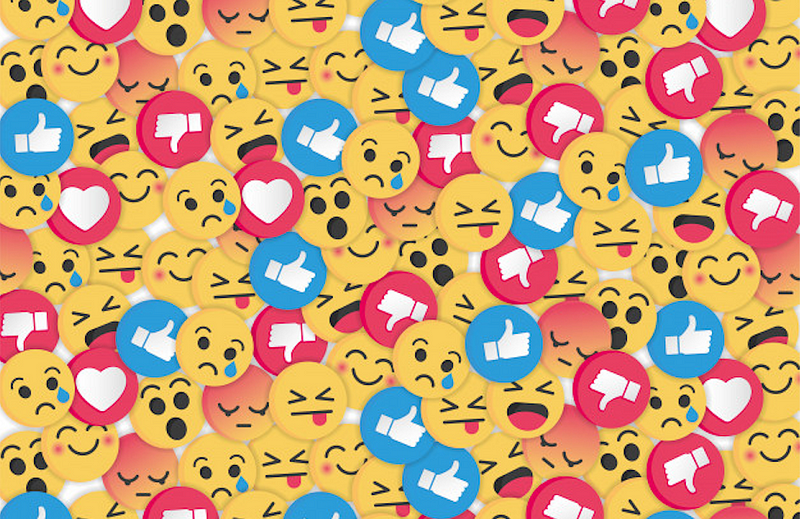
However, Clegg glosses over the origins of these signals. While he highlights the user’s Feed, he overlooks the significant influence of data generated by others. Facebook employs a method known as collaborative filtering, which helps recommend content based on the preferences of users with similar tastes. As noted in a 2015 blog post by Facebook’s engineering team, this technique helps users discover relevant content that goes beyond their immediate likes or searches.
Section 1.2: The Role of Collaborative Filtering
Clegg's diagram also lacks mention of the broader user base's influence on the algorithm. He details that the ranking process relies on posts from friends, pages, and groups, excluding content removed for violating community standards. Yet, the vast pool of Facebook's users beyond one’s direct network is conspicuously absent from this discussion.
Chapter 2: The Dilemma of User Empowerment
Instead of reducing the algorithm's sway over user feeds, Clegg introduces a feature called Favorites. This function aims to help users curate their experience by identifying the friends and pages deemed most significant to them. However, the question remains: what if users prioritize sensational content that they personally find meaningful? Clegg does not clarify how the algorithm would respond in such scenarios, raising concerns about the balance between personalized experiences and community standards.
The first video titled "Small YouTubers: Do this and the algorithm will love you" explores strategies for smaller creators to thrive on the platform. It discusses the nuances of algorithm engagement and audience connection.
The second video, "THE ALGORITHM CAN BE AGAINST YOU & YET GIVE YOU VIEWS! - YouTube," delves into the contradictory nature of algorithms, examining how they can both hinder and help content visibility.
Ultimately, Clegg’s arguments against the influence of filter bubbles, citing studies from reputable institutions, suggest that social media filters do not operate as previously believed. However, the real issue may not lie in what they exclude, but rather in what they promote.
The problem with social platform filters isn’t just about exclusion; it’s about the implications of what they allow in.
Clegg's anecdote about a bear and her cubs serves as a distraction from the underlying issues. The core problem with Facebook is its persistent recommendation system, which operates on the belief that the best suggestions come from users with similar profiles. This model has fueled its success but also its tendency to disseminate harmful content. While users may enjoy the platform's tailored experiences, the broader impact on society remains a pressing concern.