Understanding Traffic Jams: Insights from Physics and Data
Written on
Chapter 1: The Science of Traffic Jams
Have you ever been caught in a traffic jam and wished that drivers around you were more skilled? German physicists Kai Nagel and Michael Schreckenberg made a significant discovery in unraveling the complexities of traffic congestion. Surprisingly, traffic jams are not random occurrences; they can be anticipated with remarkable accuracy.

In the Nagel-Schreckenberg model, vehicles are represented as discrete entities on a grid. Each time interval allows for updates in their positions based on four straightforward principles:
- Speed Limit: Vehicles operate within a speed range from 0 to the designated limit. In the original model, this limit is set at '5', meaning vehicles can travel anywhere from a complete stop to the maximum speed of 5.
- Acceleration: Each vehicle can increase its speed by 1 at each time step, provided it remains under the speed limit.
- Collision Avoidance: If a vehicle's speed would result in a collision with the one ahead, it must decelerate. For instance, if a car is three cells behind another with a speed of 4, it will slow down to 2 to prevent overlap.
- Random Deceleration: At each time step, there's a probability, denoted as 'p', that a vehicle will reduce its speed by 1. If p equals 0.5, then each vehicle has a 50% chance of slowing down at every interval. This randomness reflects the imperfections inherent in human driving, which can lead to traffic jams.
In the accompanying video, you can observe the movement of vehicles, highlighting the formation of traffic jams (indicated by red vehicles) along with periods of quick movement (shown in green). A density of 0.35 signifies that 35% of the grid's cells are occupied by cars. For a grid of 100 cells, 35 would be filled with vehicles.
Chapter 2: Visualizing Traffic Patterns
One effective method for illustrating traffic flow is through a space-time plot. Here, dark regions represent waves of vehicle density. The negative slope demonstrates how a traffic jam originating upstream at time t=0 propagates downstream, affecting locations further along the route.
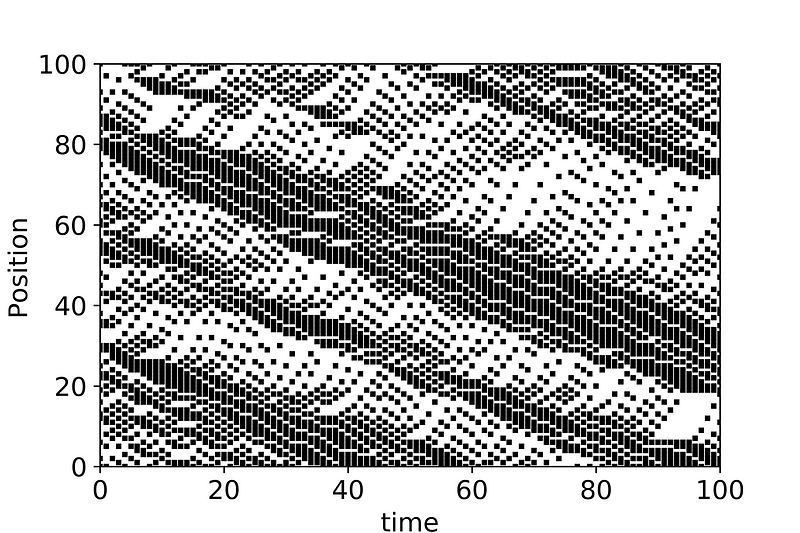
The Genesis of Traffic Jams
The relationship between vehicle density and speed reveals crucial insights into traffic behavior. As the number of cars increases, their speed tends to decrease. Once density surpasses approximately 0.2, there's a significant drop in velocity. This is because, at this density, the average distance between vehicles becomes 5, which corresponds to the maximum speed. Thus, as vehicles begin to feel the impact of those in front, they must slow down.
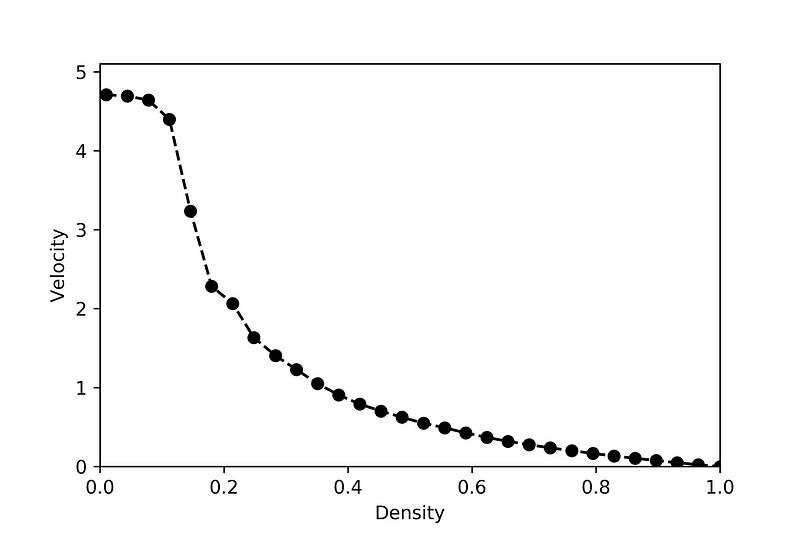
Understanding Vehicle Flux
Another valuable way to comprehend the emergence of traffic jams is through a flux-density graph. Flux indicates the number of vehicles passing a point in a set timeframe. Initially, at low densities, all vehicles can maintain the speed limit, leading to a linear increase in flux. However, as density rises, vehicles can't sustain these speeds, and a certain threshold (density = 0.2) results in decreased flux.
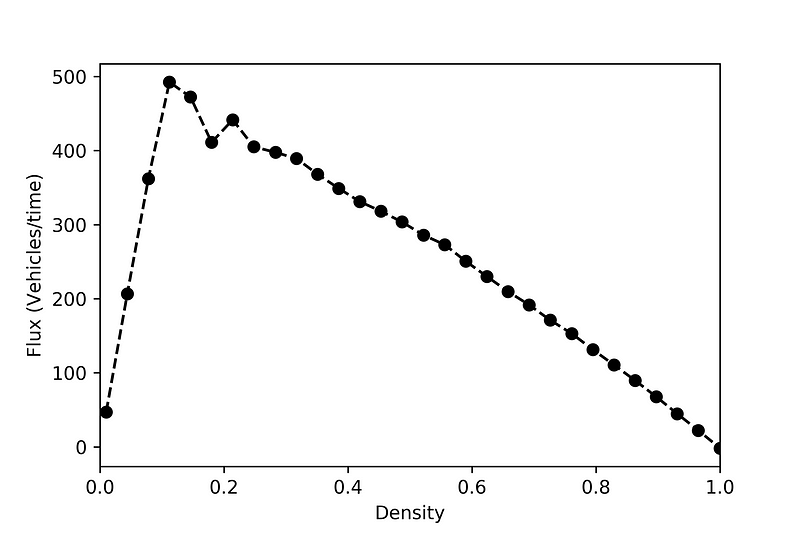
Real-World Traffic Insights
The Nagel-Schreckenberg model effectively captures fundamental traffic behaviors, but how does this translate to real-world scenarios? On June 15, 2005, researchers from the NGSIM project gathered detailed vehicle trajectory data from the southbound lanes of US 101, also known as the Hollywood Freeway in Los Angeles.
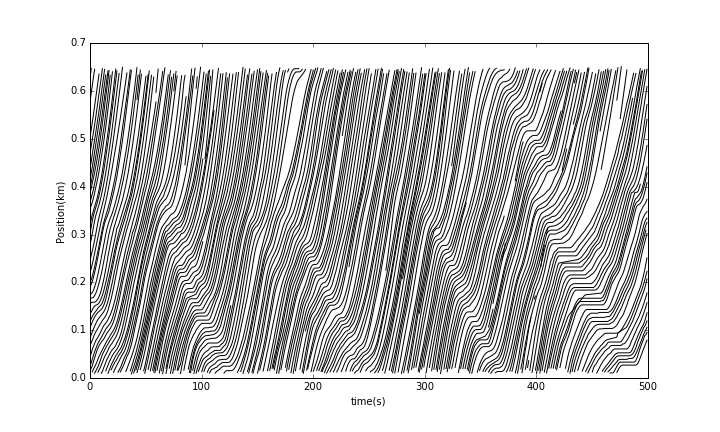
The space-time plots derived from this data showcase the distinct waves associated with traffic congestion. Much like the model, a jam that forms 0.5 km upstream eventually affects locations further downstream after approximately 100 seconds.
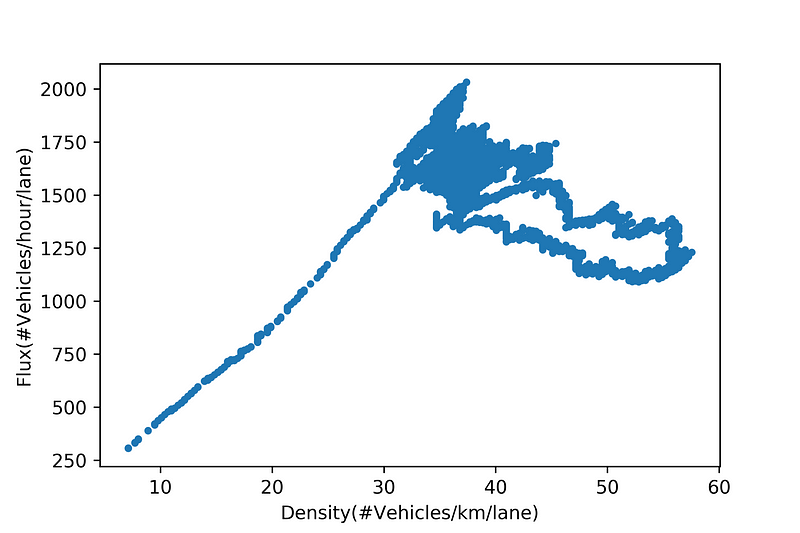
The flux-density graph from this data resembles the Nagel-Schreckenberg results, but with different units. In real-world conditions, flux starts to decline at a density of about 30 vehicles per kilometer per lane. This density is crucial because it corresponds to an average of 33 meters between vehicles when traveling at 65 mph (30 m/s), leaving just about one second for a driver to react if the vehicle ahead suddenly stops.
Conclusion
In summary, traffic jams arise due to human driving imperfections. Key factors include the tendency for erratic driving and the finite reaction time that leads us to maintain a safe distance from the car in front—typically around one second. When vehicle numbers increase, drivers find themselves uncomfortably close to one another, and their driving behavior shifts from what it would be on an open highway. So, the next time you find yourself in a traffic jam, remember to reflect on the human behaviors that contribute to it.
If you're curious about how autonomous vehicles could potentially mitigate traffic congestion, consider exploring my article: Can Autonomous Vehicles Avoid Traffic Jams?