Exploring the Parallels Between Biological Brains and ANNs
Written on
Chapter 1: Understanding Neural Mechanisms
In discussions with various individuals—most lacking expertise in neuroscience, biophysics, computational biology, or artificial intelligence (my background includes the latter four)—I often encounter the belief that artificial neural networks (ANNs) and human brains do not operate on a fundamentally similar 'neural network' principle. I wanted to share insights from my research interaction with ChatGPT, highlighting the substantial evidence supporting my perspective that both systems fundamentally function on comparable principles.
The Evidence
A wealth of research indicates that biological neural networks strive to minimize prediction errors, akin to the operations of ANNs. This idea is central to predictive coding theory, which suggests that the brain perpetually generates and refines predictions regarding incoming sensory information, adjusting its internal models to reconcile the difference between expected and actual input.
Here are several significant findings that support this concept:
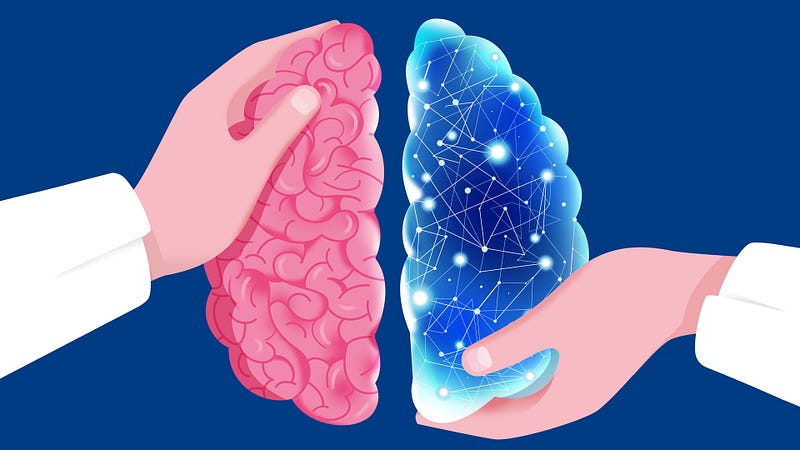
Neural Evidence
Mismatch Negativity (MMN): ERP Studies
Event-related potential (ERP) research has revealed a distinct signal known as mismatch negativity (MMN), which arises when there is a deviation from anticipated sensory input. MMN is particularly evident in response to unexpected auditory stimuli and serves as direct evidence of prediction error signaling within the brain.
Error-Related Negativity (ERN): EEG Studies
Electroencephalography (EEG) research has discovered an error-related negativity (ERN) signal that emerges when people err. This signal is believed to reflect the brain's capacity to detect prediction errors during cognitive tasks.
Predictive Signals in Sensory Cortex: fMRI and Single-Unit Recording
Studies utilizing functional MRI (fMRI) and single-unit recording have demonstrated that neurons in sensory cortices, such as those associated with vision and hearing, transmit predictive signals influenced by expectations and prior experiences. These predictive signals diminish when stimuli meet expectations and become more pronounced when there is a deviation.
Computational and Theoretical Models
Predictive Coding Models: Hierarchical Models
Predictive coding models propose a hierarchical organization of the brain, where higher levels generate predictions and lower levels encode prediction errors. These models effectively explain numerous neural phenomena, including the response characteristics of neurons in sensory cortices.
Bayesian Inference
Many models leverage Bayesian principles, allowing the brain to update its predictions based on new sensory information, thereby minimizing prediction errors over time.
Experimental Evidence
Sensory Adaptation: Visual and Auditory Systems
Experiments indicate that sensory systems adjust to environmental regularities, resulting in reduced neural responses to predictable stimuli while enhancing reactions to unexpected stimuli. This adaptive behavior can be interpreted as the brain minimizing prediction errors by aligning with the statistical properties of sensory input.
Expectation and Perception: Behavioral Studies
Behavioral research has shown that expectations can significantly influence perception. For example, individuals demonstrate quicker and more accurate responses to stimuli that align with their expectations, indicating the brain's use of predictions to optimize sensory processing.
Surprise and Learning: Reinforcement Learning
Studies in reinforcement learning reveal that unexpected outcomes lead to larger prediction errors, prompting behavioral and learning adjustments. This aligns with the notion that the brain employs prediction errors to refine its internal models and enhance future predictions.
Key Research Studies and Findings
Friston’s Free Energy Principle
Neuroscientist Karl Friston has formulated the free energy principle, which expands on predictive coding concepts. This principle posits that the brain minimizes a quantity known as "free energy," closely related to prediction error, offering a comprehensive framework for comprehending brain function through the lens of prediction error minimization.
Neural Responses to Expected vs. Unexpected Stimuli: Studies by Rao and Ballard (1999)
Groundbreaking research by Rao and Ballard illustrated that neurons in the visual cortex respond differently to expected and unexpected stimuli, reinforcing the predictive coding framework.
Hawkins’ Memory-Prediction Framework
Jeff Hawkins proposed that the neocortex is essentially a predictive machine, perpetually generating and refining predictions about sensory inputs based on memory and learning.
Conclusion
The evidence supporting the idea that biological neural networks aim to minimize prediction errors is extensive and diverse. It encompasses neural, computational, and experimental studies, demonstrating that the brain utilizes predictive coding mechanisms to enhance perception, learning, and behavior. Ongoing research continues to unveil the foundational principles governing brain function and cognition.
Moreover, these principles parallel what ANNs achieve, as the neuron node weights are mathematically adjusted to minimize prediction errors.
Chapter 2: Neural Networks in Depth
The video "How Do Neural Networks Grow Smarter? - with Robin Hiesinger" delves into the mechanisms behind the intelligence of neural networks, exploring how they evolve to perform complex tasks.
In "Three times artificial neural networks are nothing like the human brain (+ are they ever alike?)", the discussion revolves around the differences and similarities between artificial neural networks and human cognitive processes, providing insights into their distinct functionalities.